
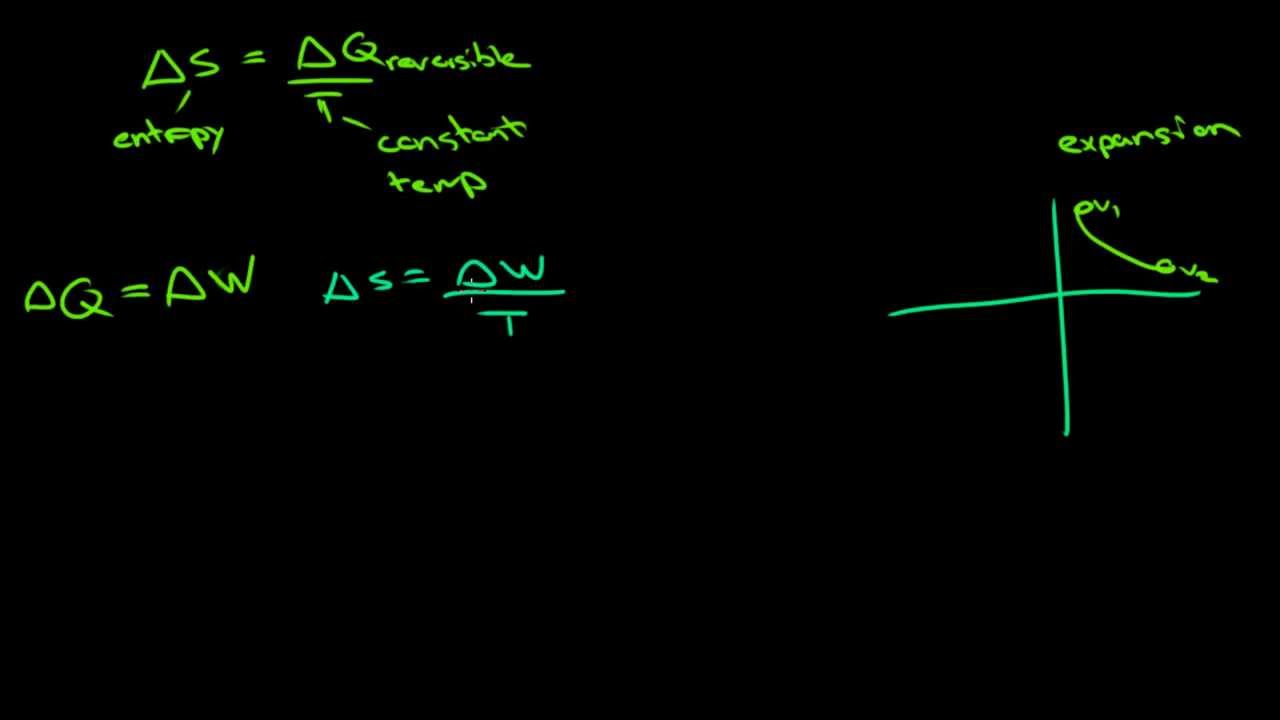
The statements you are about to read were worked out over a period of many years, were refined and clarified by countless minds, and were gradually combined into one large idea that we now recognize as a comment on the fundamental behavior of the universe. The Second Law of Thermodynamics is a statement of the painfully obvious that is, at least it's obvious to us now. Anyone who says otherwise is trying to con you. This waste can be reduced, but it can never be eliminated. Waste energy is associated with all processes. That's the point of machine learning.Discussion introduction Thermal pollution from a nuclear power plant near Perry, Ohio From the other side, we have just used a subset of combinations (14 examples) to train our algorithm (by building a decision tree) and now it can classify all other combinations without our help. Of course you can, but even for this small example, the total number of combinations is 3*2*2*3=36. You might wonder why we need a decision tree if we can just provide the decision for each combination of attributes. Now we can conclude that the first split on the "Windy" attribute was a really bad idea, and the given training examples suggest that we should test on the "Outlook" attribute first. Using this approach, we can find the information gain for each of the attributes, and find out that the "Outlook" attribute gives us the greatest information gain, 0.247 bits. the set of training examples of T such for which attribute a is equal to v the entropy of T conditioned on a ( Conditional entropy) a set of training examples, each of the form where is the value of the attribute or feature of example and is the corresponding class label, The general formula for the information gain for the attribute a is Hence the information gain as reduction in entropy is
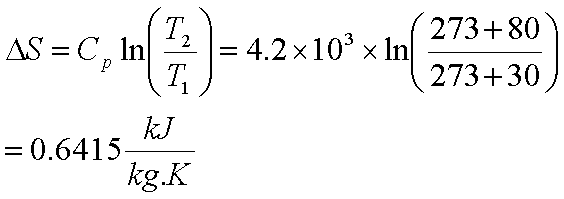
Thus, our initial entropy is 0.94, and the average entropy after the split on the "Windy" attribute is 0.892. So, the average entropy after the split would be

We have six examples with a "True" value of the "Windy" attribute and eight examples with a "False" value of the "Windy" attribute. In order to estimate entropy reduction in general, we need to average using the probability to get "True" and "False" attribute values. This is, of course, better than our initial 0.94 bits of entropy (if we are lucky enough to get "False" in our example under test). Six of them have "Yes" as the Play label, and two of them have "No" as the Play label. Now, if the value of the "Windy" attribute is "False", we are left with eight examples. So, if our example under test has "True" as the "Windy" attribute, we are left with more uncertainty than before. Three of them have "Yes" as the Play label, and three of them have "No" as the Play label. If the value of the "Windy" attribute is "True", we are left with six examples. Technically, we are performing a split on the "Windy" attribute. We decide to test the "Windy" attribute first. Now, let's imagine we want to classify an example. According to the well-known Shannon Entropy formula, the current entropy is In our training set we have five examples labelled as "No" and nine examples labelled as "Yes". How do we measure the information that each attribute can give us? One of the ways is to measure the reduction in entropy, and this is exactly what Information Gain metric does. So, by analyzing the attributes one by one, the algorithm should effectively answer the question: "Should we play tennis?" Thus, in order to perform as few steps as possible, we need to choose the best decision attribute on each step – the one that gives us maximum information. Let's look at the calculator's default data. The paths from root to leaf represent classification rules. whether a coin flip comes up heads or tails), each branch represents the outcome of the test, and each leaf node represents a class label (the decision taken after computing all the attributes). A decision tree is a flowchart-like structure in which each internal node represents a "test" on an attribute (e.g. Information gain is a metric that is particularly useful in building decision trees.
